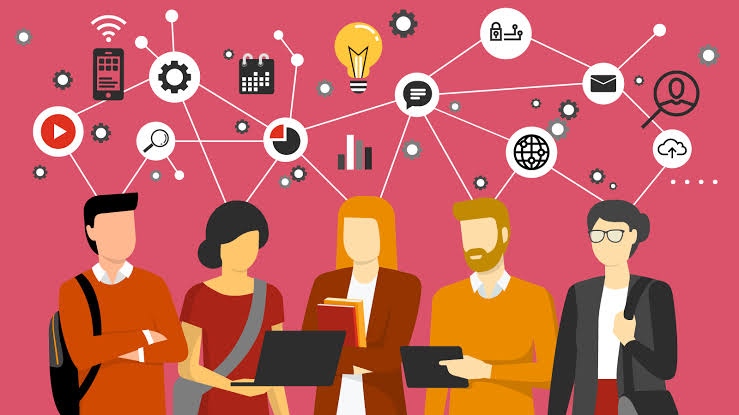
Powering Data Science with Design Thinking
Design and data science might not immediately seem like natural companions; one is associated with art and creativity while the other is a rigorous, quantitative discipline but the combination of Design Thinking and Data Science is a powerful one, but they can be fused on deriving and driving new sources of value and actionable outcomes.
Data Science seeks the variables that yield better patterns, trends, relationships, and associations operations while Design Thinking uncovers the analytical algorithm buried in the organization’s data pool and drives the subsequent adoption of the analytics.
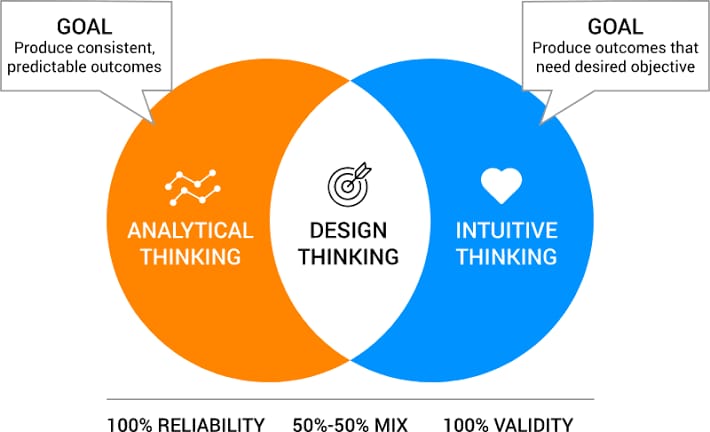
According to a report by McKinsey, organizations that blend Design Thinking with Data Science are having more success – not only in the quality and accuracy of their analytic models, but equally important in the subsequent organizational alignment around and adoption of the analytic results.
While Data Science discovers the criteria for success buried in the data (codifying trends, patterns, and relationships) , Design Thinking on the other hand discovers the criteria for success buried in the human interactions.
The first stage of the design thinking process is to build empathy with the users in order to gain a deep understanding of the user journey, motivations and consequent behaviors that are relevant to a design problem. Having built empathy with the customers and gaining an understanding of the reasons for their behaviors, businesses can then define the nature and extent of their problems more concretely.
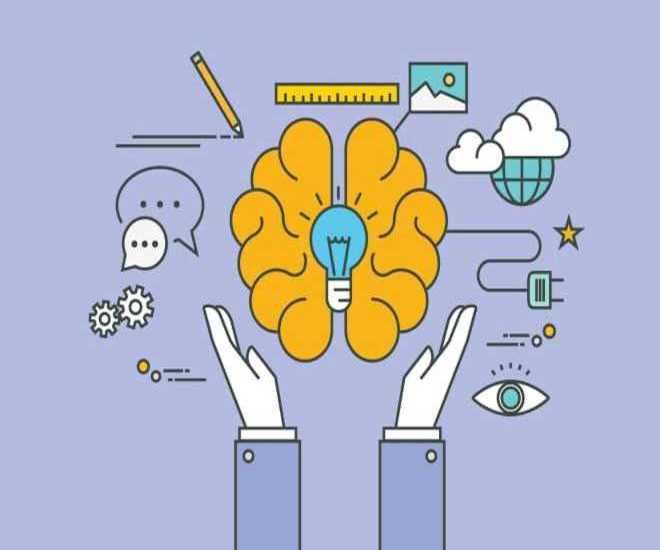
Once the information is synthesized to identify patterns, key insights that emerge from this process can be combined with well understood theory of how the world works with unexpected findings from the design research.
The clear definition of user needs enables the formation of one or more hypotheses that serve as starting points for innovative designs that address those needs in new or unexpected ways. Businesses uses data science as a critical tool for evaluating the quality of the hypotheses that they develop by being systematic about data acquisition and analysis, so that hypotheses can be directly tested against quantitative evidence to establish their comparative strengths and prioritize them accordingly.
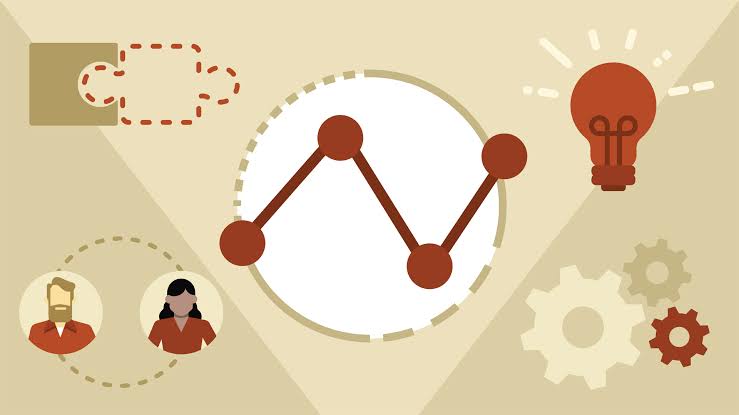
The next stage is prototyping stage where the concepts come to life. Sketching out mockup screens and product features, building user journeys and interactive prototypes, developing business models and product roadmaps to maximize product uptake and revenue. Prototypes undergo extensive iteration to ensure that they have fully addressed the insightful points uncovered during research. In design research projects, it is critical that the prototypes being developed solve the most important problems, and in the right order.
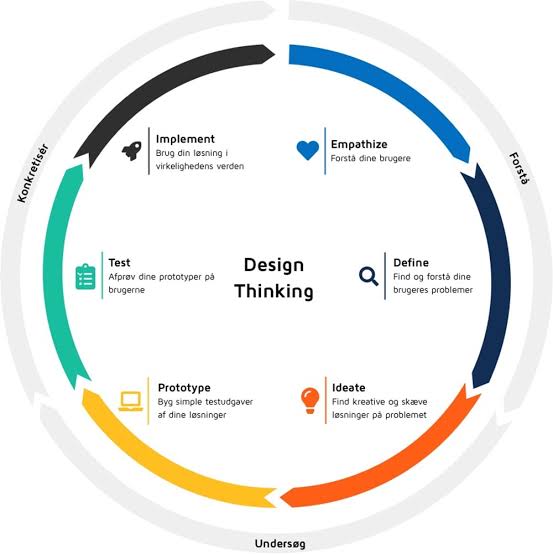
The behavioral model can be queried to ensure this is the case and to guide the improvement of the user journey. The product roadmap should include a targeted data acquisition strategy, enabling more sophisticated functionalities to be validated by user interactions before being deployed more widely and lastly comes the testing stage, for a design projects, the concept of a “test and learn” loop is useful to emphasize the iterative and ongoing nature of product refinement and improvement.
While there are classes of models that work well for small groups of users given sufficient domain knowledge, all data science techniques work better at scale. Data science provides the means to measure and compare our designs in an unbiased way. This can be done at different levels of design fidelity and at different resolutions of the model, from the granular feature level all the way up to the level of the overarching system, depending on the resources available for prototyping and testing design elements.
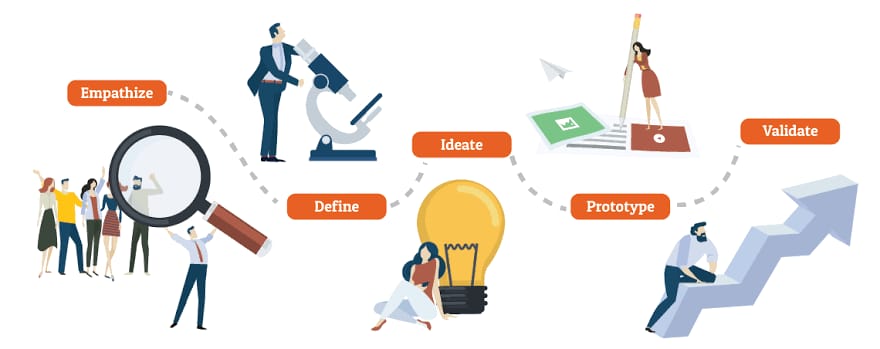
By carefully interweaving design thinking, strategy and data science, businesses can achieve design solutions that are not only conceptually and experientially outstanding, but that will also succeed in the marketplace. At the end of the day, the organizations that would win will be the ones that learn how to leverage Data Science and Design Thinking to drive the business and operational focus, and align, adopt and monetize these enabling technology combinations. The principles of the design process or design thinking, can help get the most out of data science, by providing a methodology for dealing with the ambiguous and complex nature of the data science models. It can also help in ensuring that the resulting insights are actionable and valuable for stakeholders, who remain at the core of the process.